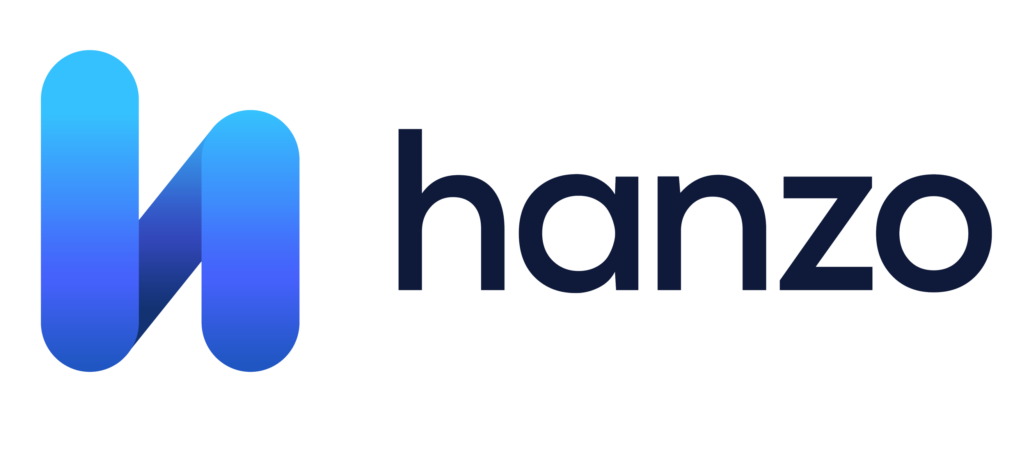
Extract from Aidan Randle-Conde’s article “Part I: Navigating AI Success Metrics – Precision and Recall in Email and Document Analysis”
Introduction
In our first post, we will dive into the world of email datasets. Emails are a critical component of business communication, and AI plays a significant role in managing, sorting, and even responding to them. We will explore how precision and recall can be applied to document-based datasets to evaluate the effectiveness of AI in handling email communications. This post is designed to set a strong foundation for understanding how to measure AI success in processing and analyzing written content.
AI metrics in legal document analysis
In the legal sector, there are many times when we might want to take a corpus of documents and split them into different categories, such as finding responsive content for first-pass review. This is typically a long and laborious process to complete by hand. Many tools are out there that can help with these classification tasks, and it can be useful to compare how they perform.
The challenge of classifying legal documents
Suppose you have a corpus of emails and a definition of a “responsive” document, with all other documents being “non-responsive.” Let’s say that the corpus has 1,100 documents in total, and 100 of them are responsive, but you don’t know which ones they are. You set your team to work to classify the documents into “Relevant” and “Not Relevant” and get the results shown below.