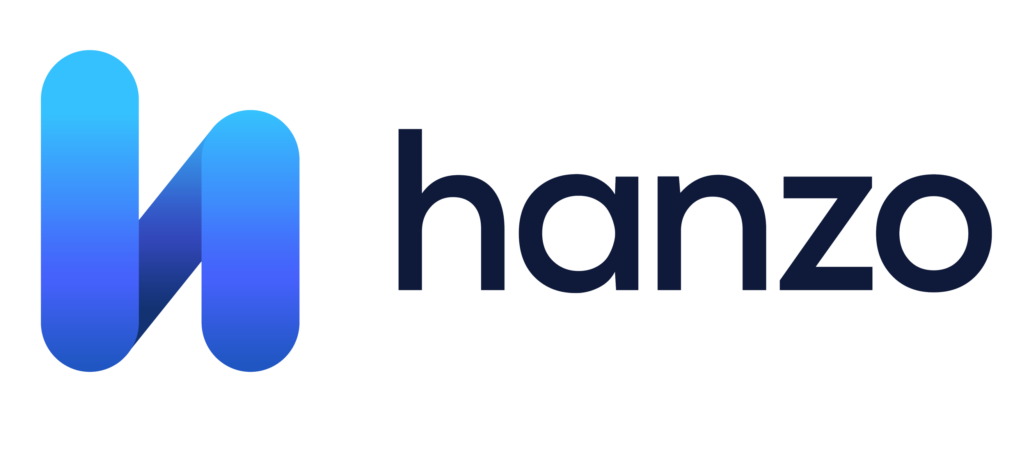
Extract from Aidan Randle-Conde’s article “Part II: Navigating AI Success Metrics – Understanding Conversational Data”
Introduction
Welcome to Part II of our series on Navigating AI Success Metrics, where we’ll dive into the nuances of understanding conversational data with AI. After laying the groundwork in Part I with the core concepts of Recall, Rejection, and Precision, we’re now turning our attention to the dynamic and often complex world of conversational data, like those found in Slack or Microsoft Teams interactions. The conversations held within these platforms are goldmines of insight, requiring sophisticated approaches to measure relevance. But first, a little review of the terms.
Recall, rejection, and precision: Key concepts revisited
In Part I of this series on Navigating AI Success Metrics”, we introduced the terms Recall, Rejection, and Precision, using first-pass review of emails as an example:
- Recall: The fraction of relevant documents correctly identified as “Responsive”.
- Rejection: The fraction of not relevant documents correctly identified as “Not Responsive.”
- Precision: The fraction of documents identified as “Responsive” that are relevant.
We found that, in general, Recall and Rejection are in tension with each other, making it difficult to develop a process that achieves high values for both.