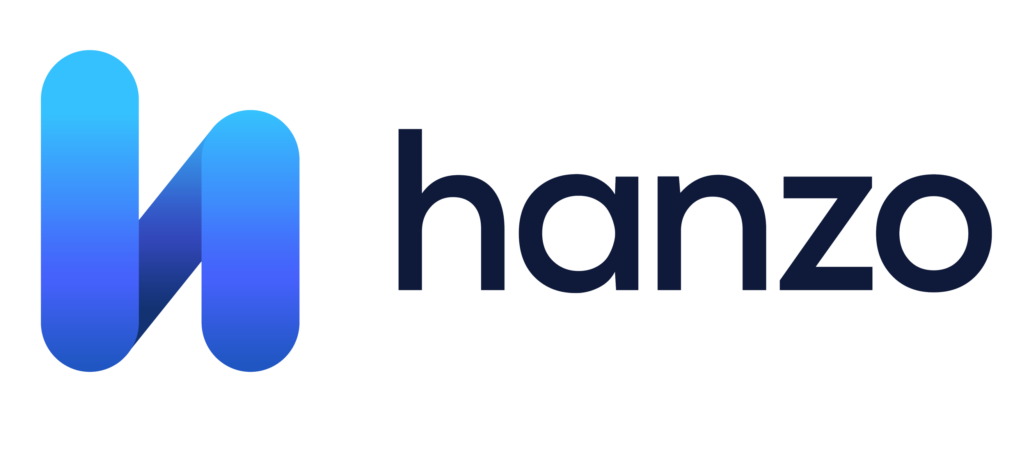
Extract from Aidan Randle-Conde’s article “Part II: Optimizing eDiscovery with AI — Enhancing Transparency”
Recalling our discussion in Part 1 on data security and cost management, this second installment focuses on the critical element of transparency in using LLMs. Understanding how AI tools derive their outputs is essential for legal professionals to trust and effectively use the technology. We will discuss how Hanzo ensures that users are fully informed about the workings and outputs of LLMs, enhancing reliability and reducing the risk of errors.
Understanding LLMs and Their Training Data
LLMs are trained on huge “foundational datasets” of public data. These foundational datasets include (but are not limited to) all of Wikipedia, the works of Shakespeare, billions of public web pages, legal and financial documents, and other content. When LLMs generate content, they can draw on patterns from these foundational datasets to decide what text to generate. This means that when using common LLM tools in today’s marketplace and asking the LLM to generate content, it can generate text based on what we ask it and the content of the foundational dataset.
The Problem of Hallucinations in LLMs
When an LLM generates text based on the user’s input and the foundational dataset, this process can lead to “hallucinations,” where the LLM produces text that is either factually incorrect or irrelevant to the query. Such hallucinations can present significant risks in the legal industry, as they can result in misleading or inaccurate information being considered credible, often without adequate verification.