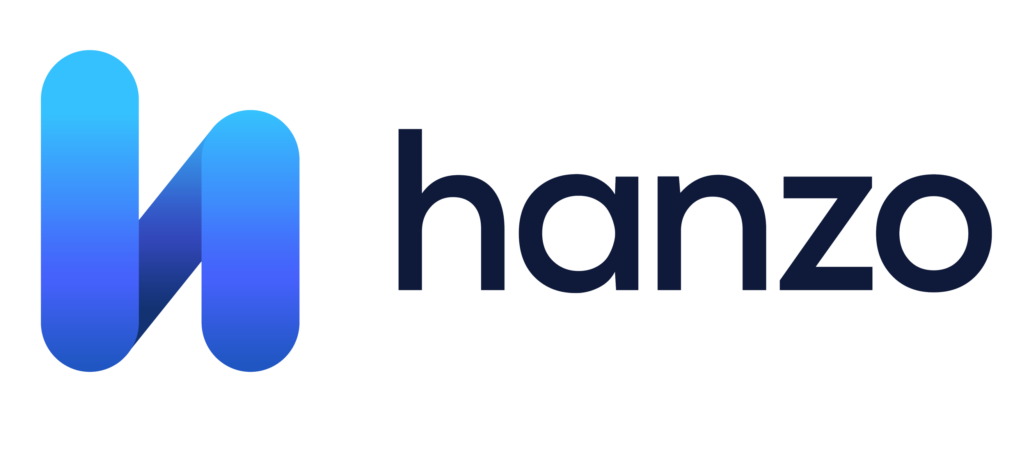
Extract from Aidan Randle-Conde’s article “Part III: Navigating AI Success Metrics – Bringing It All Together”
Introduction to mixed data in legal review
In Parts I and II, we tackled the basics of applying Recall, Rejection, and Precision in evaluating AI’s performance on document and conversational datasets. Part III brings these threads together for legal professionals seeking to enhance eDiscovery and investigation processes with AI. In Part III, we’ll navigate the complexities of combining emails and conversational data, the practicalities of using Large Language Models (LLMs), and rethink our definitions of “Document” and “Recall” to fit this mixed data landscape. We want to demystify how these advanced tools can be tailored to the unique needs of legal review and investigatory workflows.
Understanding the data landscape
With emails, we can work with a single document that arrives at one time and contains much information. This makes it simple to define Recall, Rejection, and Precision and to use TAR and CAL to speed up classification. However, when we add conversational data to the mix, we must change how we define documents to include surrounding messages and think about discussions instead of messages. This provides a challenge for TAR and CAL, as we need to define a new type of document, a group of messages, and any definition comes with its own problems. Do we group messages together in chunks of time? If so, we will have many orphan messages that appear on their own. If we group messages consecutively, we will have adjacent messages that could be hours or days apart. Whichever way we choose to split the conversations up, sometimes a discussion will be split across two or more chunks of messages. There are also differences between typical values for Recall and Precision between emails and conversational data, with emails typically having higher Recall but lower Precision and conversational data typically having lower Recall but higher Precision. When combining different datasets, it’s useful to keep track of the multiple values for Recall or Precision to reflect the different natures of the datasets.