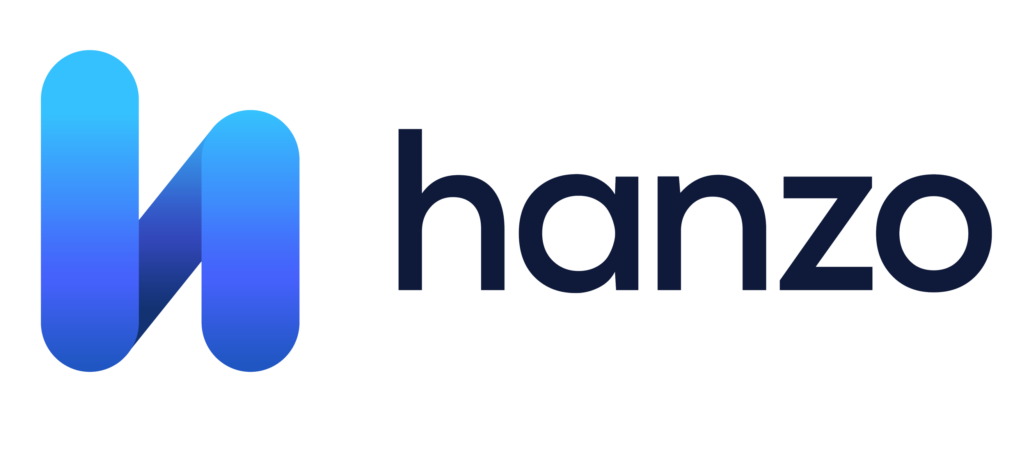
Extract from Aidan Randle-Conde’s article “Part III: Optimizing eDiscovery with AI — Overcoming Scaling Challenges”
Recap and Introduction to Scaling Challenges
In previous blog posts, we discussed how large language models (LLMs) can be used in eDiscovery and the importance of data security, keeping costs low, and transparency of data analysis. One of the novel risks introduced by using LLMs is “hallucinations,” or when an LLM generates inaccurate or irrelevant text. In this post, we will put everything together and tackle the challenge of scale.
Meeting Multiple Requirements with LLMs
As we have seen, Hanzo effectively meets several critical requirements in the deployment of Large Language Models (LLMs) for legal eDiscovery:
- Data security: Datasets are segregated safely in customer environments to maintain a single-tenant policy. This method minimizes the risk of data breaches and unauthorized access, providing a robust security framework that is essential in handling sensitive legal information.
- Cost: To manage costs effectively, Hanzo utilizes LLMs in a targeted manner. By deploying the smallest appropriate model for each specific task, Hanzo ensures that computational resources are used efficiently. Furthermore, the operational model is designed so that machines remain active only for the time needed to complete the task, significantly reducing unnecessary expenditures on processing power and energy.