The next decade will witness the introduction of AI regulation at both the Federal and State level. Much of this regulation will focus on the use of autonomous and self-driving cars. In 2016 the Obama administration introduced a report on the future of artificial intelligence. The report summarized the status of AI in American society, economy, and government. It looked at specific applications, such as transportation and war fighting, and makes a series of nonbinding recommendations. This was followed in 2018 by the Trump administration’s Summit on Artificial Intelligence for American Industry, reviewing the Trump administration’s regulatory and policy to AI.
On the 10th of April 2019, Congress made its first legislative foray into the issue of algorithmic governance with the Algorithmic Accountability Act of 2019. This was a first attempt at addressing the issue of AI regulation and in many ways appeared to miss the mark. The bill holds algorithms to different standards than humans, without consideration for the non-linear nature of software development. It also primarily targets large firms despite the clear potential for smaller organizations to cause harm. Unsurprisingly the bill did not get very far. However, both the contents of the bill and recent congressional and senate inquiries into tech has shown that lawmakers are currently not equipped to write effective legislation that covers issues which are complex, important, and rapidly evolving. Further research is needed by congress to understand how algorithms work before legislation is adopted which embraces the precautionary principle.
The initial problem with the Algorithmic Accountability act is its framing. Targeting only automated high-risk decision-making, rather than all high-risk decision-making, is counterproductive. To hold algorithmic decisions to a higher standard than human decisions implies that automated decisions are inherently less trustworthy or more dangerous than human ones, which is not the case. This would only serve to stigmatize and discourage the use of AI solutions, reducing social benefits and the US share of a what is estimated to be a $15.7 trillion industry by 2030.
Other proposals include industry reports by private firms and multinational organizations. In February 2020, the European Commission had published a white paper presenting policy options to enable a trustworthy and secure development of AI in Europe, in full respect of the values and rights of EU citizens. The main building blocks of this report include a policy framework setting our measures to align the efforts of member states, in partnership with the public and private sector. In addition, the report focuses heavily of developing an ecosystem of trust to support a human-centric approach based on human-centric values, including the development of agreed upon ethical guidelines for current and future policy development.
Central to these initiatives are questions surrounding the ethical use of data. There have been several suggestions for determining the key features of healthy AI policy. Some of these include human agency and oversight; technical robustness and safety; privacy and data governance; transparency; diversity, non-discrimination, and fairness; societal and environmental wellbeing; and accountability. Whilst some countries have begun the process of setting strict standards around the access to and ethical use of data, regulations surrounding the application of image recognition and classification is yet to be written.
It is important to understand not only where image classification AI is used, but also how it works. After all, the applications go beyond unlocking the latest iPhone. Image classification AI can find existing application in document review. Examples include identifying documents containing specific logo’s, separating our schematics or patent data, and identifying oil field maps and relevant imagery.
Your brain is constantly processing data that you have observed from the world around you. You absorb the data, you make predictions about the data, and then you act upon them. In vision, a receptive field of a single sensory neuron is the specific region of the retina in which something will affect the firing of that neuron (i.e., it activates). Every sensory neuron cell has similar receptive fields, and their fields are overlying. When light hits the retina (a light-sensitive layer of tissue at the back of the eye), special cells called photoreceptors turn the light into electrical signals. These electrical signals travel from the retina through the optic nerve to the brain. Then the brain turns the signals into the images you see.
It is important to note that the concept of hierarchy plays a significant role in the brain. The neocortex stores information hierarchically, in cortical columns, or groupings of uniformly organized neurons in the neocortex. 1980, a hierarchical neural network model called the neocognitron was proposed by Kunihiko Fukushima, which was inspired by the concept of simple and complex cells. This would form the foundations of further research toward the development of image classification AI.
Pretty simple stuff, right? Well, not really. So how about we reframe the idea and kick the anatomy lesson to the side so we can think about this as if we are the computers. When we see an object, we label it based on what we have already learned in the past. Essentially, we have trained on data over a long period of time so that buildings, cards, people, and other objects are all clearly classified according to their respective group. The world is training data. Our eyes are the input function, and between the eyes and brain is a series of dials which process and fine tune the data into a final, recognizable image in our brain. If you have never seen a car before, you have no training data to refer to. That data includes the height, width, and depth of the physical world. It also includes colors, shadows, and movement. This is where the inspiration for image classification AI models originates.
Convolutional neural networks (CNNs) are a subfield of deep learning. Remember AlphaGo? Deep learning (also referred to as deep neural networks) is a subfield of machine learning. What makes deep learning unique is the way it imitates the working of the human brain in processing data and creating patterns for use in decision making. Theses artificial neural networks utilize neuron nodes that are connected like a web in layers between an input layer and output layer. While traditional programs build analysis with data in a linear way, the hierarchical function of deep learning systems enable machines to process data with a nonlinear approach.
CNN’s have a different architecture than regular neural nets. Regular neural nets transform an input by putting it through a series of hidden layers. Every layer is made up of a set of neurons, where each layer is fully connected to all neurons in the layer before. Finally, there is a last fully-connected layer – the output layer – that represents the predictions.
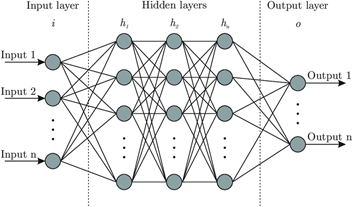
CNN’s are a bit different. For starters, the layers are organized in three dimensions: width, height, and depth. Further, the neurons in one layer do not connect to all the neurons in the next layer but only to a small region of it. Lastly, the final output will be reduced to a single vector of probability scores, organized along the depth dimension.
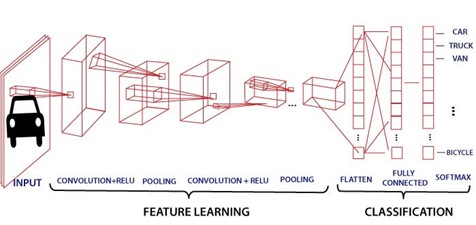
In CNNs, the computer visualizes an input image as an array of pixels. The better the image resolution, the better the training data. The purpose of CNN’s is to enable machines to view the world and perceive it as close to the way humans do. The applications are broad, including image & video recognition, image analysis and classification, media recognition, recommendation systems, and natural language processing (among others).
The CNN has two components: feature extraction and classification. The process starts with the convolutional layer, which extracts features from the input image. This preserves the relationship between pixels by learning features using small squares of input data. The convolution of an image with different filters can perform operations such as edge detection, blur and sharpen by applying filters. Next come the strides, or the number of pixels shifting over the input matrix. If you had a picture of a tiger, this is the part where the network would recognize its teeth, its stripes, two ears, and four legs. During classification, connected layers of neurons serve as a classifier on top of the extracted features. Each layer assigns a probability for the object on the image being a tiger.
This technology is not new, it has been utilized by tech firms for years to capture and understand data. Today many of us utilize CNN’s to unlock our phones through facial recognition security features. CNN’s have increased application as a security measure at borders, through customs, and within law enforcement for identifying people of interest. There are serious ethical and legal concerns surrounding the bias of training data and violation of PII. More broadly, what do appropriate regulatory measures look like to prevent potential abuse? Whatever format future legislation looks like, an increase in professional education remains more critical than ever. There are powerful social and economic forces at work that could prove detrimental should regulatory policy be instituted that seeks to blame the algorithm and not the context of its use.
Artificial Intelligence regulation is not just complex terrain, it is uncharted territory in an age that is passing the baton from human leadership to machine learning emergence, automation, robotic manufacturing, and deep learning reliance. Artificial Intelligence is largely seen as a commercial tool, but it is quickly becoming an ethical dilemma for the internet with the rise of AI forgery and a new breed of content in which it is more difficult to detect what is real and what is not real online. Artificial Intelligence regulation that is too aggressive will fall behind, with serious economic and security consequences. Instead, we should focus on specific applications of Artificial Intelligence and regulate based on the performance of the function, not how it is achieved. Autonomous cards should be regulated as cards; they should safely deliver users to their destinations in the real world, and overall reduce the number of accidents; how they achieve this is irrelevant.