Image classification AI can find existing application in document review. Examples include identifying documents containing specific logo’s, separating our schematics or patent data, and identifying oil field maps and relevant imagery. The sophistication of legal AI solutions has grown to include progressively more pragmatic image classification solutions for both litigation and compliance functions. The next year or so will see more thought leadership toward the application of image classification tools, and the shift from early adopters to the more pragmatic side of the market. Once the technology has aligned with delivery, courts will begin to begin to expect higher standards of sophistication from litigation teams in utilizing these tools. With that in mind, let’s review image classification AI, so we might understand not only what image classification AI is, but how it works.
Your brain is constantly processing data that you have observed from the world around you. You absorb the data, you make predictions about the data, and then you act upon them. In vision, a receptive field of a single sensory neuron is the specific region of the retina in which something will affect the firing of that neuron (i.e., it activates). Every sensory neuron cell has similar receptive fields, and their fields are overlying. When light hits the retina (a light-sensitive layer of tissue at the back of the eye), special cells called photoreceptors turn the light into electrical signals. These electrical signals travel from the retina through the optic nerve to the brain. Then the brain turns the signals into the images you see.
It is important to note that the concept of hierarchy plays a significant role in the brain. The neocortex stores information hierarchically, in cortical columns, or groupings of uniformly organized neurons in the neocortex. 1980, a hierarchical neural network model called the neocognitron was proposed by Kunihiko Fukushima, which was inspired by the concept of simple and complex cells. This would form the foundations of further research toward the development of image classification AI.
Pretty simple stuff, right? Well, not really. So how about we reframe the idea and kick the anatomy lesson to the side so we can think about this as if we are the computers. When we see an object, we label it based on what we have already learned in the past. Essentially, we have trained on data over a long period of time so that buildings, cards, people, and other objects are all clearly classified according to their respective group. The world is training data. Our eyes are the input function, and between the eyes and brain is a series of dials which process and fine tune the data into a final, recognizable image in our brain. If you have never seen a car before, you have no training data to refer to. That data includes the height, width, and depth of the physical world. It also includes colors, shadows, and movement. This is where the inspiration for image classification AI models originates.
Convolutional neural networks (CNNs) are a subfield of deep learning. Remember AlphaGo? Deep learning (also referred to as deep neural networks) is a subfield of machine learning. What makes deep learning unique is the way it imitates the working of the human brain in processing data and creating patterns for use in decision making. Theses artificial neural networks utilize neuron nodes that are connected like a web in layers between an input layer and output layer. While traditional programs build analysis with data in a linear way, the hierarchical function of deep learning systems enable machines to process data with a nonlinear approach.
CNN’s have a different architecture than regular neural nets. Regular neural nets transform an input by putting it through a series of hidden layers. Every layer is made up of a set of neurons, where each layer is fully connected to all neurons in the layer before. Finally, there is a last fully-connected layer – the output layer – that represents the predictions.
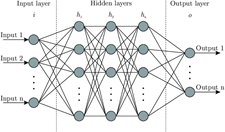
CNN’s are a bit different. For starters, the layers are organized in three dimensions: width, height, and depth. Further, the neurons in one layer do not connect to all the neurons in the next layer but only to a small region of it. Lastly, the final output will be reduced to a single vector of probability scores, organized along the depth dimension.
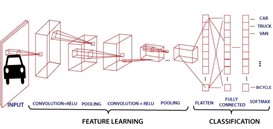
In CNNs, the computer visualizes an input image as an array of pixels. The better the image resolution, the better the training data. The purpose of CNN’s is to enable machines to view the world and perceive it as close to the way humans do. The applications are broad, including image & video recognition, image analysis and classification, media recognition, recommendation systems, and natural language processing (among others).
The CNN has two components: feature extraction and classification. The process starts with the convolutional layer, which extracts features from the input image. This preserves the relationship between pixels by learning features using small squares of input data. The convolution of an image with different filters can perform operations such as edge detection, blur and sharpen by applying filters. Next come the strides, or the number of pixels shifting over the input matrix. If you had a picture of a tiger, this is the part where the network would recognize its teeth, its stripes, two ears, and four legs. During classification, connected layers of neurons serve as a classifier on top of the extracted features. Each layer assigns a probability for the object on the image being a tiger.
Leading service providers will see this technology as an opportunity to grow service capabilities in other areas of the legal domain, such as compliance monitoring, contracts, and meeting regulatory standards. More broadly, as law enforcement gradually introduces this technology over time, court expectations will move alongside. Currently there are limitations to how certain agencies and business functions can leverage image classification AI, but it is prime for widespread adoption within the eDiscovery space as firms harness these algorithms to provide image learning review solutions to their customers. This is a game changer for firms on the cutting edge, as it opens the potential for new revenue streams in potentially more profitable applications.